I recently came across a bug that causes intermittent connection resets. After some digging, I found it was caused by a subtle combination of several different network subsystems. It helped me understand Kubernetes networking better, and I think it’s worthwhile to share with a wider audience who are interested in the same topic.
The symptom
We received a user report claiming they were getting connection resets while using a Kubernetes service of type ClusterIP to serve large files to pods running in the same cluster. Initial debugging of the cluster did not yield anything interesting: network connectivity was fine and downloading the files did not hit any issues. However, when we ran the workload in parallel across many clients, we were able to reproduce the problem. Adding to the mystery was the fact that the problem could not be reproduced when the workload was run using VMs without Kubernetes. The problem, which could be easily reproduced by a simple app, clearly has something to do with Kubernetes networking, but what?
Kubernetes networking basics
Before digging into this problem, let’s talk a little bit about some basics of Kubernetes networking, as Kubernetes handles network traffic from a pod very differently depending on different destinations.
Pod-to-Pod
In Kubernetes, every pod has its own IP address. The benefit is that the applications running inside pods could use their canonical port, instead of remapping to a different random port. Pods have L3 connectivity between each other. They can ping each other, and send TCP or UDP packets to each other. CNI is the standard that solves this problem for containers running on different hosts. There are tons of different plugins that support CNI.
Pod-to-external
For the traffic that goes from pod to external addresses, Kubernetes simply uses SNAT. What it does is replace the pod’s internal source IP:port with the host’s IP:port. When the return packet comes back to the host, it rewrites the pod’s IP:port as the destination and sends it back to the original pod. The whole process is transparent to the original pod, who doesn’t know the address translation at all.
Pod-to-Service
Pods are mortal. Most likely, people want reliable service. Otherwise, it’s pretty much useless. So Kubernetes has this concept called “service” which is simply a L4 load balancer in front of pods. There are several different types of services. The most basic type is called ClusterIP. For this type of service, it has a unique VIP address that is only routable inside the cluster.
The component in Kubernetes that implements this feature is called kube-proxy. It sits on every node, and programs complicated iptables rules to do all kinds of filtering and NAT between pods and services. If you go to a Kubernetes node and type iptables-save
, you’ll see the rules that are inserted by Kubernetes or other programs. The most important chains are KUBE-SERVICES
, KUBE-SVC-*
and KUBE-SEP-*
.
KUBE-SERVICES
is the entry point for service packets. What it does is to match the destination IP:port and dispatch the packet to the correspondingKUBE-SVC-*
chain.KUBE-SVC-*
chain acts as a load balancer, and distributes the packet toKUBE-SEP-*
chain equally. EveryKUBE-SVC-*
has the same number ofKUBE-SEP-*
chains as the number of endpoints behind it.KUBE-SEP-*
chain represents a Service EndPoint. It simply does DNAT, replacing service IP:port with pod’s endpoint IP:Port.
For DNAT, conntrack kicks in and tracks the connection state using a state machine. The state is needed because it needs to remember the destination address it changed to, and changed it back when the returning packet came back. Iptables could also rely on the conntrack state (ctstate) to decide the destiny of a packet. Those 4 conntrack states are especially important:
- NEW: conntrack knows nothing about this packet, which happens when the SYN packet is received.
- ESTABLISHED: conntrack knows the packet belongs to an established connection, which happens after handshake is complete.
- RELATED: The packet doesn’t belong to any connection, but it is affiliated to another connection, which is especially useful for protocols like FTP.
- INVALID: Something is wrong with the packet, and conntrack doesn’t know how to deal with it. This state plays a centric role in this Kubernetes issue.
Here is a diagram of how a TCP connection works between pod and service. The sequence of events are:
- Client pod from left hand side sends a packet to a service: 192.168.0.2:80
- The packet is going through iptables rules in client node and the destination is changed to pod IP, 10.0.1.2:80
- Server pod handles the packet and sends back a packet with destination 10.0.0.2
- The packet is going back to the client node, conntrack recognizes the packet and rewrites the source address back to 192.169.0.2:80
- Client pod receives the response packet
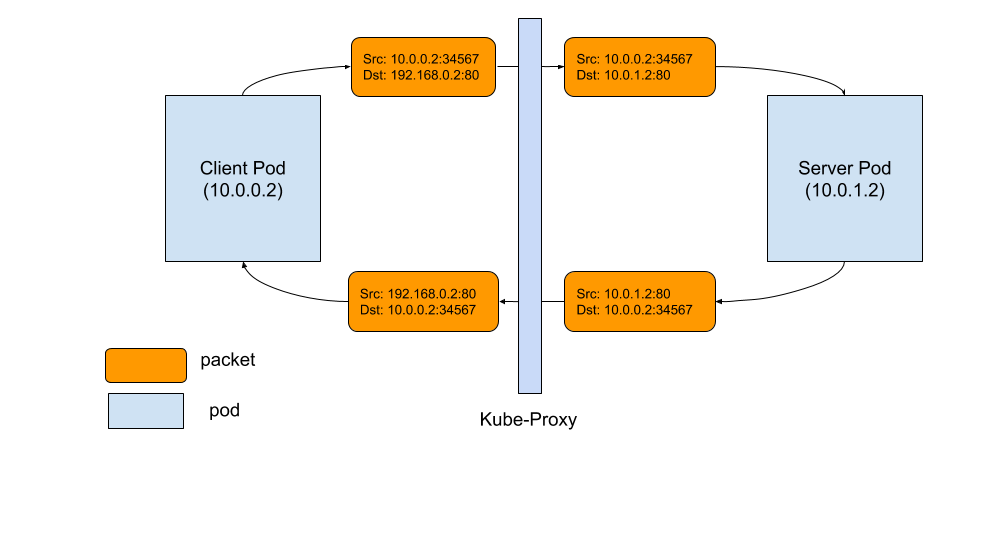
What caused the connection reset?
Enough of the background, so what really went wrong and caused the unexpected connection reset?
As the diagram below shows, the problem is packet 3. When conntrack cannot recognize a returning packet, and mark it as INVALID. The most common reasons include: conntrack cannot keep track of a connection because it is out of capacity, the packet itself is out of a TCP window, etc. For those packets that have been marked as INVALID state by conntrack, we don’t have the iptables rule to drop it, so it will be forwarded to client pod, with source IP address not rewritten (as shown in packet 4)! Client pod doesn’t recognize this packet because it has a different source IP, which is pod IP, not service IP. As a result, client pod says, “Wait a second, I don’t recall this connection to this IP ever existed, why does this dude keep sending this packet to me?” Basically, what the client does is simply send a RST packet to the server pod IP, which is packet 5. Unfortunately, this is a totally legit pod-to-pod packet, which can be delivered to server pod. Server pod doesn’t know all the address translations that happened on the client side. From its view, packet 5 is a totally legit packet, like packet 2 and 3. All server pod knows is, “Well, client pod doesn’t want to talk to me, so let’s close the connection!” Boom! Of course, in order for all these to happen, the RST packet has to be legit too, with the right TCP sequence number, etc. But when it happens, both parties agree to close the connection.
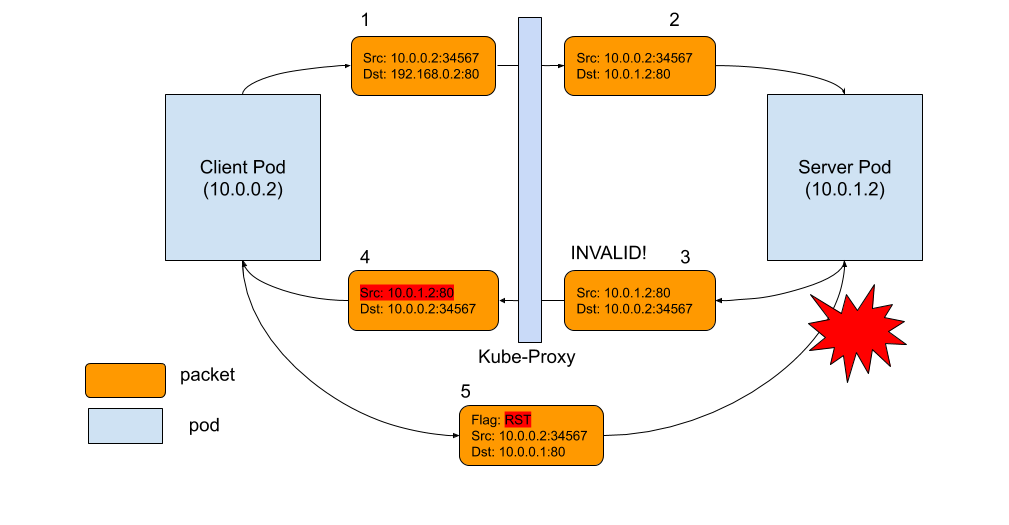
How to address it?
Once we understand the root cause, the fix is not hard. There are at least 2 ways to address it.
- Make conntrack more liberal on packets, and don’t mark the packets as INVALID. In Linux, you can do this by
echo 1 > /proc/sys/net/ipv4/netfilter/ip_conntrack_tcp_be_liberal
. - Specifically add an iptables rule to drop the packets that are marked as INVALID, so it won’t reach to client pod and cause harm.
The fix is drafted (https://github.com/kubernetes/kubernetes/pull/74840), but unfortunately it didn’t catch the v1.14 release window. However, for the users that are affected by this bug, there is a way to mitigate the problem by applying the following rule in your cluster.
apiVersion: extensions/v1beta1
kind: DaemonSet
metadata:
name: startup-script
labels:
app: startup-script
spec:
template:
metadata:
labels:
app: startup-script
spec:
hostPID: true
containers:
- name: startup-script
image: gcr.io/google-containers/startup-script:v1
imagePullPolicy: IfNotPresent
securityContext:
privileged: true
env:
- name: STARTUP_SCRIPT
value: |
#! /bin/bash
echo 1 > /proc/sys/net/ipv4/netfilter/ip_conntrack_tcp_be_liberal
echo done
Summary
Obviously, the bug has existed almost forever. I am surprised that it hasn’t been noticed until recently. I believe the reasons could be: (1) this happens more in a congested server serving large payloads, which might not be a common use case; (2) the application layer handles the retry to be tolerant of this kind of reset. Anyways, regardless of how fast Kubernetes has been growing, it’s still a young project. There are no other secrets than listening closely to customers’ feedback, not taking anything for granted but digging deep, we can make it the best platform to run applications.