Sep 19, 2018
by José Moreira
The approach for deploying a containerised application typically involves building a Docker container image from a Dockerfile once and deploying that same image across several deployment environments (development, staging, testing, production).
If following security best practices, each deployment environment will require a different configuration (data storage authentication credentials, external API URLs) and the configuration is injected into the application inside the container through environment variables or configuration files. Our Hamish Hutchings takes a deeper look at 12-factor app in this blog post. Also, the possible configuration profiles might not be predetermined, if for example, the web application should be ready to be deployed both on public or private cloud (client premises), as it is also common for several configuration profiles to be added to source code and the required profile to be loaded at build time.
The structure of a web application project typically contains a ‘src’ directory with source code and executing npm run-script build triggers the Webpack asset build pipeline. Final asset bundles (HTML, JS, CSS, graphics, and fonts) are written to a dist directory and contents are either uploaded to a CDN or served with a web server (NGINX, Apache, Caddy, etc).
For context in this article, let’s assume the web application is a single-page frontend application which connects to a backend REST API to fetch data, for which the API endpoint will change across deployment environments. The backend API endpoint should, therefore, be fully configurable and configuration approach should support both server deployment and local development and assets are served by NGINX.
Deploying client-side web application containers requires a different configuration strategy compared to server-side applications containers. Given the nature of client-side web applications, there is no native executable that can read environment variables or configuration files in runtime, the runtime is the client-side web browser and configuration has to be hard-coded in the Javascript source code either by hard-coding values during the asset build phase or hard-coding rules (a rule would be to deduce current environment based on the domain name, ex: ‘staging.app.com’).
There is one OS process which is relevant to configuration in which reading values from environment values is useful, which is the asset build Node JS process and this is helpful for configuring the app for local development with local development auto reload.
For the configuration of the webapp across several different environments, there are a few solutions:
- Rebuild the Webpack assets on container start during each deployment with the proper configuration on the destination server node(s):
- Adds to deployment time. Depending on deployment rate and size of the project, the deployment time overhead might be considerable.
- Is prone to build failures at end of deployment pipeline even if the image build has been tested before.
- Build phase can fail for example due to network conditions although this can probably be minimised by building on top of a docker image that already has all the dependencies installed.
- Might affect rollback speed
- Build one image per environment (again with hardcoded configuration):
- Similar solutions (and downsides) of solution #1 except that it adds clutter to the docker registry/daemon.
- Build image once and rewrite configuration bits only during each deployment to target environment:
- Image is built once and ran everywhere. Aligns with the configuration pattern of other types of applications which is good for normalisation
- Scripts that rewrite configuration inside the container can be prone to failure too but they are testable.
I believe solution #1 is viable and, in some cases, simpler and probably required if the root path where the web application is hosted needs to change dynamically, ex.: from ‘/’ to ‘/app’, as build pipelines can hardcode the base path of fonts and other graphics in CSS files with the root path, which is a lot harder to change post build.
Solution #3 is the approach I have been implementing for the projects where I have been responsible for containerising web applications (both at my current and previous roles), which is the solution also implemented by my friend Israel and for which he helped me out implementing the first time around and the approach that will be described in this article.
Application-level configuration
Although it has a few moving parts, the plan for solution #3 is rather straightforward:

For code samples, I will utilise my fork of Adam Sandor micro-service Doom web client, which I have been refactoring to follow this technique, which is a Vue.js application. The web client communicates with two micro-services through HTTP APIs, the state and the engine, endpoints which I would like to be configurable without rebuilding the assets.
Single Page Applications (SPA) have a single “index.html” as a entry point to the app and during deployment. meta tags with optional configuration defaults are added to the markup from which application can read configuration values. script tags would also work but I found meta tags simple enough for key value pairs.
1
2
3
4
5
6
7
8
9
10
11
12
13
14
15
16
17
18
19
20
21
|
<!DOCTYPE html>
<html lang=”en”>
<head>
<meta charset=”utf-8″>
<meta http-equiv=”X-UA-Compatible” content=”IE=edge”>
<meta name=”viewport” content=”width=device-width,initial-scale=1.0″>
<meta property=”DOOM_STATE_SERVICE_URL” content=”http://localhost:8081/” />
<meta property=”DOOM_ENGINE_SERVICE_URL” content=”http://localhost:8082/” />
<link rel=”icon” href=”./favicon.ico”>
<title>frontend</title>
</head>
<body>
<noscript>
<strong>We’re sorry but frontend doesn’t work properly without JavaScript enabled. Please enable it to continue.</strong>
</noscript>
<div id=”app”></div>
<!– built files will be auto injected –>
</body>
</html>
|
For reading configuration values from meta tags (and other sources), I wrote a simple Javascript module (“/src/config.loader.js”):
1
2
3
4
5
6
7
8
9
10
11
12
13
14
15
16
17
18
19
20
21
22
23
24
25
26
27
28
29
30
31
|
/**
* Get config value with precedence:
* – check `process.env`
* – check current web page meta tags
* @param key Configuration key name
*/
function getConfigValue (key) {
let value = null
if (process.env && process.env[`$`] !== undefined) {
// get env var value
value = process.env[`$`]
} else {
// get value from meta tag
return getMetaValue(key)
}
return value
}
/**
* Get value from HTML meta tag
*/
function getMetaValue (key) {
let value = null
const node = document.querySelector(`meta[property=$]`)
if (node !== null) {
value = node.content
}
return value
}
export default { getConfigValue, getMetaValue }
|
This module will read configuration “keys” by looking them up in the available environment variables (“process.env”) first, so that configuration can be overridden with environment variables when developing locally (webpack dev server) and then the current document meta tags.
I also abstracted the configuration layer by adding a “src/config/index.js” that exports an object with the proper values:
|
import loader from ‘./loader’
export default {
DOOM_STATE_SERVICE_URL: loader.getConfigValue(‘DOOM_STATE_SERVICE_URL’),
DOOM_ENGINE_SERVICE_URL: loader.getConfigValue(‘DOOM_ENGINE_SERVICE_URL’)
}
|
which can then be utilised in the main application by importing the “src/config” module and accessing the configuration keys transparently:
|
import config from ‘./config’
console.log(config.DOOM_ENGINE_SERVICE_URL)
|
There is some room for improvement in the current code as it not DRY (list of required configuration variables is duplicated in several places in the project) and I’ve considered writing a simple Javascript package to simplify this approach as I’m not aware if something already exists. Writing the Docker & Docker Compose files The Dockerfile for the SPA adds source-code to the container to the ‘/app’ directory, installs dependencies and runs a production webpack build (“NODE_ENV=production”). Assets bundles are written to the “/app/dist” directory of the image:
|
FROM node:8.11.4-jessie
RUN mkdir /app
WORKDIR /app
COPY package.json .
RUN npm install
COPY . .
ENV NODE_ENV production
RUN npm run build
CMD npm run dev
|
The docker image contains a Node.js script (“/app/bin/rewrite-config.js”) which copies “/app/dist” assets to another target directory before rewriting the configuration. Assets will be served by NGINX and therefore copied to a directory that NGINX can serve, in this case, a shared (persistent) volume. Source and destination directories can be defined through container environment variables:
1
2
3
4
5
6
7
8
9
10
11
12
13
14
15
16
17
18
19
20
21
22
23
24
25
26
27
28
29
30
31
32
33
34
35
36
37
38
39
40
41
42
43
44
45
46
47
48
49
50
51
52
53
54
55
56
57
58
59
|
#!/usr/bin/env node
const cheerio = require(‘cheerio’)
const copy = require(‘recursive-copy’)
const fs = require(‘fs’)
const rimraf = require(‘rimraf’)
const DIST_DIR = process.env.DIST_DIR
const WWW_DIR = process.env.WWW_DIR
const DOOM_STATE_SERVICE_URL = process.env.DOOM_STATE_SERVICE_URL
const DOOM_ENGINE_SERVICE_URL = process.env.DOOM_ENGINE_SERVICE_URL
// – Delete existing files from public directory
// – Copy `dist` assets to public directory
// – Rewrite config meta tags on public directory `index.html`
rimraf(WWW_DIR + ‘/*’, {}, function() {
copy(`$`, `$`, , function(error, results) {
if (error) {
console.error(‘Copy failed: ‘ + error);
} else {
console.info(‘Copied ‘ + results.length + ‘ files’);
rewriteIndexHTML(`$/index.html`, {
DOOM_STATE_SERVICE_URL: DOOM_STATE_SERVICE_URL,
DOOM_ENGINE_SERVICE_URL: DOOM_ENGINE_SERVICE_URL
})
}
});
})
/**
* Rewrite meta tag config values in “index.html”.
* @param file
* @param values
*/
function rewriteIndexHTML(file, values) {
console.info(`Reading ‘$’`)
fs.readFile(file, ‘utf8’, function (error, data) {
if (!error) {
const $ = cheerio.load(data)
console.info(`Rewriting values ‘$’`)
for (let [key, value] of Object.entries(values)) {
console.log(key, value);
$(`[property=$]`).attr(“content”, value);
}
fs.writeFile(file, $.html(), function (error) {
if (!error) {
console.info(`Wrote ‘$’`)
} else {
console.error(error)
}
});
} else {
console.error(error)
}
});
}
|
The script utilises CheerioJS to read the “index.html” into memory, replaces values of meta tags according to environment variables and overwrites “index.html”. Although “sed” would have been sufficient for search & replace, I chose CherioJS as a more reliable solution that also allows expanding into more complex solutions like script injections.
Deployment with Kubernetes
Let’s jump into the Kubernetes Deployment manifest:
1
2
3
4
5
6
7
8
9
10
11
12
13
14
15
16
17
18
19
20
21
22
23
24
25
26
27
28
29
30
31
32
33
34
35
36
37
38
39
40
41
42
43
44
45
46
47
48
49
50
|
—
# Source: doom-client/templates/deployment.yaml
apiVersion: apps/v1beta2
kind: Deployment
metadata:
name: doom-client
labels:
name: doom-client
spec:
replicas: 1
selector:
matchLabels:
name: doom-client
template:
metadata:
labels:
name: doom-client
spec:
initContainers:
– name: doom-client
image: “doom-client:latest”
command: [“/app/bin/rewrite-config.js”]
imagePullPolicy: IfNotPresent
env:
– name: DIST_DIR
value: “/app/dist”
– name: WWW_DIR
value: “/tmp/www”
– name: DOOM_ENGINE_SERVICE_URL
value: “http://localhost:8081/”
– name: DOOM_STATE_SERVICE_URL
value: “http://localhost:8082/”
volumeMounts:
– name: www-data
mountPath: /tmp/www
containers:
– name: nginx
image: nginx:1.14
imagePullPolicy: IfNotPresent
volumeMounts:
– name: www-data
mountPath: /usr/share/nginx/html
– name: doom-client-nginx-vol
mountPath: /etc/nginx/conf.d
volumes:
– name: www-data
emptyDir: {}
– name: doom-client-nginx-vol
configMap:
name: doom-client-nginx
|
The Deployment manifest defines an “initContainer” which executes the “rewrite-config.js” Node.js script to prepare and update the shared storage volume with the asset bundles. It also defines an NGINX container for serving our static assets. Finally, it also creates a shared Persistent Volume which is mounted on both of the above containers. In the NGINX container the mount point is “/var/www/share/html” but on the frontend container “/tmp/www” for avoiding creating extra directories. “/tmp/www” will be the directory where the Node.js script will copy asset bundles to and rewrite the “index.html”. Local development with Docker Compose
The final piece of our puzzle is the local Docker Compose development environment. I’ve included several services that allow both developing the web application with the development server and testing the application when serving production static assets through NGINX. It is perfectly possible to separate these services into several YAML files (“docker-compose.yaml”, “docker-compose.dev.yaml” and “docker-compose.prod.yaml”) and do some composition but I’ve added a single file for the sake of simplicity.
Apart from the “doom-state” and “doom-engine” services which are our backend APIs, the “ui” service starts the webpack development server with “npm run dev” and the “ui-deployment” service, which runs a container based on the same Dockerfile, runs the configuration deployment script. The “nginx” service serves static assets from a persistent volume (“www-data”) which is also mounted on the “ui-deployment” script.
1
2
3
4
5
6
7
8
9
10
11
12
13
14
15
16
17
18
19
20
21
22
23
24
25
26
27
28
29
30
31
32
33
34
35
36
37
38
39
40
41
42
43
44
45
46
47
48
49
50
51
52
53
54
55
56
57
58
59
60
61
62
63
64
|
# docker-compose.yaml
version: ‘3’
services:
ui:
build: .
command: [“npm”, “run”, “dev”, ]
ports:
– “8080:8080”
environment:
– HOST=0.0.0.0
– PORT=8080
– NODE_ENV=development
– DOOM_ENGINE_SERVICE_URL=http://localhost:8081/
– DOOM_STATE_SERVICE_URL=http://localhost:8082/
volumes:
– .:/app
# bind volume inside container for source mount not shadow image dirs
– /app/node_modules
– /app/dist
doom-engine:
image: microservice-doom/doom-engine:latest
environment:
– DOOM_STATE_SERVICE_URL=http://doom-state:8080/
– DOOM_STATE_SERVICE_PASSWORD=enginepwd
ports:
– “8081:8080”
doom-state:
image: microservice-doom/doom-state:latest
ports:
– “8082:8080”
# run production deployment script
ui-deployment:
build: .
command: [“/app/bin/rewrite-config.js”]
environment:
– NODE_ENV=production
– DIST_DIR=/app/dist
– WWW_DIR=/tmp/www
– DOOM_ENGINE_SERVICE_URL=http://localhost:8081/
– DOOM_STATE_SERVICE_URL=http://localhost:8082/
volumes:
– .:/app
# bind volume inside container for source mount not shadow image dirs
– /app/node_modules
– /app/dist
# shared NGINX static files dir
– www-data:/tmp/www
depends_on:
– nginx
# serve docker image production build with nginx
nginx:
image: nginx:1.14
ports:
– “8090:80”
volumes:
– www-data:/usr/share/nginx/html
volumes:
www-data:
|
Since the webpack dev server is a long running process which also hot-reloads the app on source code changes, the Node.js config module will yield configuration from environment variables, based on the precedence I created. Also, although source code changes can trigger client-side updates without restarts (hot reload), it will not update the production build, which has to be manual but straightforward with a $ docker-compose build && docker-compose up.
Summarizing, although there are a few improvements points, including on the source code I wrote for this implementation, this setup has been working pretty well for the last few projects and is flexible enough to also support deployments to CDNs, which is as simple as adding a step for pushing assets to the cloud instead of a shared volume with NGINX.
If you have any comments feel free to get in touch on Twitter or comment under the article.
Download our free whitepaper, Kubernetes: Crossing the Chasm below.
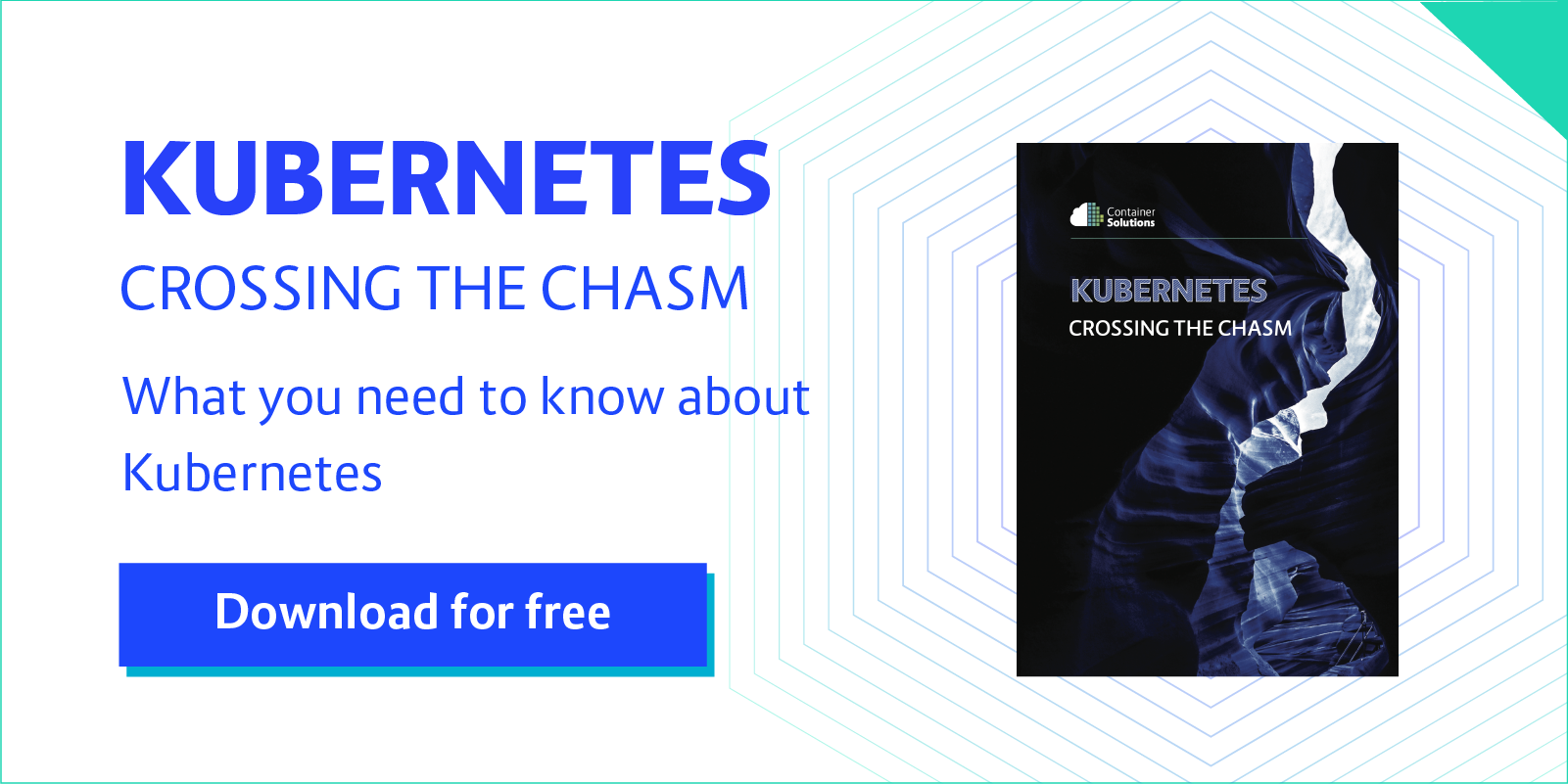
Source