By Scott McCarty February 22, 2018July 16, 2018
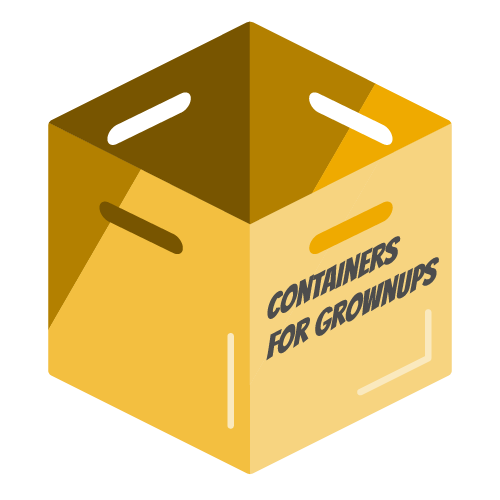
You might think containers seem like a pretty straightforward concept, so why do I need to read about container terminology? In my work as a container technology evangelist, I’ve encountered misuse of container terminology that causes people to stumble on the road to mastering containers. Terms like containers and images are used interchangeably, but there are important conceptual differences. In the world of containers, repository has a different meaning than what you’d expect. Additionally, the landscape for container technologies is larger than just docker. Without a good handle on the terminology, It can be difficult to grasp the key differences between docker and (pick your favorites, CRI-O, rkt, lxc/lxd) or understand what the Open Container Initiative is doing to standardize container technology.
It is deceptively simple to get started with Linux Containers. It takes only a few minutes to install a container engine like docker and run your first commands. Within another few minutes, you are building your first container image and sharing it. Next, you begin the familiar process of architecting a production-like container environment, and have the epiphany that it’s necessary to understand a lot of terminology and technology behind the scenes. Worse, many of the following terms are used interchangeably… often causing quite a bit of confusion for newcomers.
- Container
- Image
- Container Image
- Image Layer
- Registry
- Repository
- Tag
- Base Image
- Platform Image
- Layer
Understanding the terminology laid out in this technical dictionary will provide you a deeper understanding of the underlying technologies. This will help you and your teams speak the same language and also provide insight into how to better architect your container environment for the goals you have. As an industry and wider community, this deeper understanding will enable us to build new architectures and solutions. Note, this technical dictionary assumes that the reader already has an understanding of how to run containers. If you need a primer, try starting with A Practical Introduction to Docker Containers on the Red Hat Developer Blog.
Containers 101
To understand container terminology, it’s important to understand exactly what a container is – with technical precision. A container is really two different things. Like a normal Linux program, containers really have two states – rest and running. When at rest, a container is a file (or set of files) that is saved on disk. This is referred to as a Container Image or Container Repository. When you type the command to start a container, the Container Engine unpacks the required files and meta-data, then hands them off to the the Linux kernel. Starting a container is very similar to starting a normal Linux process and requires making an API call to the Linux kernel. This API call typically initiates extra isolation and mounts a copy of the files that were in the container image. Once running, Containers are just a Linux process. The process for starting containers, as well as the image format on disk, are defined and governed by standards.
There are several competing Container Image formats (Docker, Appc, LXD), but the industry is moving forward with a standard governed under the Open Container Initiative – sometimes referred to simply as Open Containers or the OCI. The scope of the OCI includes a Container Image Format Specification, which defines the on-disk format for container images as well as the meta-data which defines things like hardware architecture and the operating system (Linux, Windows, etc). An industry wide container image format enables ecosystems of software to flourish – different individual contributors, projects, and vendors are able to build images and tooling, which are interoperable. Users want interoperability between tools for signing, scanning, building, running, moving and managing container images.
There are also several competing Container Engines including Docker, CRI-O, Railcar, RKT, LXC. These Container Engines take a Container Image and turn it into a Container (aka running processes). How this happens is governed by the scope of the OCI which includes a Container Runtime Specification and a Reference Runtime Implementation called RunC. This reference implementation is open source, governed by a community development model, and commonly used by many container engines to communicate with the host kernel when creating containers.
Tools which target the OCI Container Image Format Specification and Container Runtime Specification ensure portability between a broad ecosystem of container platforms, container engines, and supporting tools across cloud providers and on premise architectures. Understanding the nomenclature, container standards, and the architecture of the building blocks of containers, will ensure that you can communicate with other architects to build scalable & supportable containerized applications and environments to productively run containers for years to come.
Background
Containers 101
Basic Vocabulary
Container Image
Container Image Format
Container Engine
Container
Container Host
Registry Server
Container Orchestration
Advanced Vocabulary
Container Runtime
Image Layer
Tag
Repository
Namespace
Kernel Namespace
Graph Driver
Container Use Cases
Application Containers
Operating System Containers
Pet Containers
Super Privileged Containers
Tools & Operating System Software
Architecture of Containers
Application Images
Base Images
Builder Images
Containerized Components
Deployer Images
Intermediate Images
Intermodal Container Images
System Containers
Conclusion
Container Image
See also Repository.
A container image, in its simplest definition, is a file which is pulled down from a Registry Server and used locally as a mount point when starting Containers. The container community uses “container image” quite a bit, but this nomenclature can be quite confusing. Docker, RKT, and even LXD, operate on the concept of pulling remote files and running them as a Container. Each of these technologies treats container images in different ways. LXD pulls a single container image (single layer), while docker and RKT use OCI-based images which can be made up of multiple layers.
Technically, it is much more complicated than a single file on a Registry Server. When people use the term “container image,” they often mean to imply Repository and referring to a bundle of multiple container Image Layers as well as metadata which provides extra information about the layers.
Implicit in the concept of a container image is the concept of a Container Image Format.
Container Image Format
See Container Image and Background.
Historically, each Container Engine had its container images format. LXD, RKT, and Docker all had their own image formats. Some were made up of a single layer, while others were made up of a bunch of layers in a tree structure. Today, almost all major tools and engines have moved to a format defined by the Open Container Initiative (OCI).This image format defines the layers and metadata within a container image. Essentially, the OCI image format defines a container image composed of tar files for each layer, and a manifest.json file with the metadata.
The Open Container Initiative (OCI), which was originally based on the Docker V2 image format, has successfully unified a wide ecosystem of container engines, cloud providers and tools providers (security scanning, signing, building and moving). This will help protect users as the invest in knowledge, and tooling in their environments.
Container Engine
See also Container Runtime.
A container engine is a piece of software that accepts user requests, including command line options, pulls images, and from the end user’s perspective runs the container. There are many container engines, including docker, RKT, CRI-O, and LXD. Also, many cloud providers, Platforms as a Service (PaaS), and Container Platforms have their own built-in container engines which consume Docker or OCI compliant Container Images. Having an industry standard Container Image Format allows interoperability between all of these different platforms.
Going one layer deeper, most container engines don’t actually run the containers, they rely on an OCI compliant runtime like runc. Typically, the container runtime is responsible:
- Handling user input
- Handling input over an API often from a Container Orchestrator
- Pulling the Container Images from the Registry Server
- Expanding decompressing and expanding the container image on disk using a Graph Driver (block, or file depending on driver)
- Preparing a container mount point, typically on copy-on-write storage (again block or file depending on driver)
- Preparing the metadata which will be passed to the container Container Runtime to start the Container correctly
- Using some defaults from the container image (ex.ArchX86)
- Using user input to override defaults in the container image (ex. CMD, ENTRYPOINT)
- Using defaults specified by the container image (ex. SECCOM rules)
- Calling the Container Runtime
For an even deeper understanding, please see Understanding the Container Standards. See also Container Runtime.
Container
Containers have existed within operating systems for quite a long time. A container is the runtime instantiation of a Container Image. A container is a standard Linux process typically created through a clone() system call instead of fork() or exec(). Also, containers are often isolated further through the use of cgroups, SELinux or AppArmor.
Container Host
The container host is the system that runs the containerized processes, often simply called containers. This could be, for example, RHEL Atomic Host running in a VM, as an instance in the public cloud, or on bare metal in your data center. Once a container image (aka repository) is pulled from a Registry Server to the local container host, it is said to be in the local cache.
Determining which repositories are synchronized to the local cache can be done with the following command:
[root@rhel7 ~]# docker images -a
REPOSITORY TAG IMAGE ID CREATED VIRTUAL SIZE
registry.access.redhat.com/rhel7 latest 6883d5422f4e 3 weeks ago 201.7 MB
Registry Server
A registry server is essentially a fancy file server that is used to store docker repositories. Typically, the registry server is specified as a normal DNS name and optionally a port number to connect to. Much of the value in the docker ecosystem comes from the ability to push and pull repositories from registry servers.

When a docker daemon does not have a locally cached copy of a repository, it will automatically pull it from a registry server. Most Linux distributions have the docker daemon configured to pull from docker.io but it is configurable on some Linux distributions. For example, Red Hat Enterprise Linux is configured to pull repositories from registry.access.redhat.com first, then it will try docker.io (Docker Hub).
It is important to stress that there is implicit trust in the registry server. You must determine how much you trust the content provided by the registry and you may want to allow or block certain registries. In addition to security, there are other concerns such as users having access to licensed software and compliance issues. The simplicity with which docker allows users to pull software makes it critical that you trust upstream content.
In Red Hat Enterprise Linux, the default docker registry is configurable. Specific registry servers can be added or blocked in RHEL7 and RHEL7 Atomic by modifying the configuration file:
vi /etc/sysconfig/docker
In RHEL7 and RHEL 7 Atomic, Red Hat’s registry server is configured out of the box:
ADD_REGISTRY=’–add-registry registry.access.redhat.com’
As a matter of security, it may be useful to block public docker repositories such as DockerHub:
# BLOCK_REGISTRY=’–block-registry’
Red Hat also offers an integrated Registry Server with OpenShift Container Platform, a standalone enterprise Registry Server with Quay Enterprise, as well as cloud based, public and private repositories on Quay.io.
Container Orchestration
Often teams start with installing a Container Host, then pulling some Container Images. Then they move on to building some new Container Images and pushing them to a Registry Server to share with others on their team. After a while they want to wire a few containers together and deploy them as a unit. Finally, at some point, they want to push that unit into a pipeline (Dev/QA/Prod) leading towards production. This is the path towards the realization that orchestration is needed.
A container orchestrator really does two things:
- Dynamically schedules container workloads within a cluster of computers. This is often referred to as distributed computing.
- Provides a standardized application definition file (kube yaml, docker compose, etc)
The above two features provide many capabilities:
- Allows containers within an application to be scheduled completely separately. This is useful if:
- Allows the utilization of large clusters of Container Hosts
- Individual containers fail (process hang, out of memory (OOM))
- Container Hosts fail (disk, network, reboot)
- Container Engines fail (corruption, restart)
- Individual containers need to be scaled up, or scaled down
- It’s easy to deploy new instances of the same application into new environments. In a cloud native world, or traditional world, there are many reasons why you might want to do this including:
- On developers laptop with a container orchestrator running
- On a shared development environment in a private namespace
- On a shared development environment in an internally public namespace for visibility and testing
- On a quality assurance (QA) environment internally
- On a load test environment dynamically provisioned and deprovisioned in the cloud
- On a gold environment to test compatibility with production
- On a production environment
- On a disaster recovery environment
- On a new production environment which has upgraded Container Hosts, Container Engines, or container orchestrators
- On a new production environment with the same versions of Container Host, Container Engine, and container orchestrator in a new geolocation (APAC, EMEA, etc)
There are many container schedulers being developed in the community and by vendors. Historically, Swarm, Mesos, and Kubernetes were the big three, but recently even Docker and Mesosphere have announced support for Kubernetes – as has almost every major cloud service provider. Kubernetes has become the defacto standard in container orchestration, similar to Linux before it. If you are looking at container orchestration, Red Hat recommends our enterprise distribution called OpenShift.
Container Runtime
A container runtime a lower level component typically used in a Container Engine but can also be used by hand for testing. The Open Containers Initiative (OCI) Runtime Standard reference implementation is runc. This is the most widely used container runtime, but there are others OCI compliant runtimes, such as crun, railcar, and katacontainers. Docker, CRI-O, and many other Container Engines rely on runc.
The container runtime is responsible for:
- Consuming the container mount point provided by the Container Engine (can also be a plain directory for testing)
- Consuming the container metadata provided by the Container Engine (can be a also be a manually crafted config.json for testing)
- Communicating with the kernel to start containerized processes (clone system call)
- Setting up cgroups
- Setting up SELinux Policy
- Setting up App Armor rules
To provide a bit of history, when the Docker engine was first created it relied on LXC as the container runtime. Later, the Docker team developed their own library called libcontainer to start containers. This library was written in Golang, and compiled into the original Docker engines. Finally, when the OCI was created, Docker donated the libcontainer code and turned it into a standalone utility called runc. Now, runc is the reference implementation and used by other Container Engines such as CRI-O. At the lowest level, this provides the ability to start a container consistently, no matter the container engine. Runc is a very terse utility and expects a mount point (directory) and meta-data (config.json) to be provided to it. See this tutorial for more on runc.
For an even deeper understanding, please see Understanding the Container Standards. See also Container Runtime.
Image Layer
Repositories are often referred to as images or container images, but actually they are made up of one or more layers. Image layers in a repository are connected together in a parent-child relationship. Each image layer represents changes between itself and the parent layer.
Below, we are going to inspect the layers of a repository on the local container host. Since Docker 1.7, there is no native tooling to inspect image layers in a local repository (there are tools for online registries). With the help of a tool called Dockviz, you can quickly inspect all of the layers. Notice that each layer has tag and a Universally Unique Identifier (UUID). The following command will returned shortened versions of the UUID that are typically unique enough to work with on a single machine. If you need to the full UUID, use the –no-trunc option.
docker run –rm –privileged -v /var/run/docker.sock:/var/run/docker.sock nate/dockviz images -t
├─2332d8973c93 Virtual Size: 187.7 MB
│ └─ea358092da77 Virtual Size: 187.9 MB
│ └─a467a7c6794f Virtual Size: 187.9 MB
│ └─ca4d7b1b9a51 Virtual Size: 187.9 MB
│ └─4084976dd96d Virtual Size: 384.2 MB
│ └─943128b20e28 Virtual Size: 386.7 MB
│ └─db20cc018f56 Virtual Size: 386.7 MB
│ └─45b3c59b9130 Virtual Size: 398.2 MB
│ └─91275de1a5d7 Virtual Size: 422.8 MB
│ └─e7a97058d51f Virtual Size: 422.8 MB
│ └─d5c963edfcb2 Virtual Size: 422.8 MB
│ └─5cfc0ce98e02 Virtual Size: 422.8 MB
│ └─7728f71a4bcd Virtual Size: 422.8 MB
│ └─0542f67da01b Virtual Size: 422.8 MB Tags: docker.io/registry:latest
Notice, that the “docker.io/registry” repository is actually made up of many images layers. More importantly, notice that a user could potentially “run” a container based off of any one of these layers. The following command is perfectly valid, though not guaranteed to have been tested or actually even work correctly. Typically, an image builder will tag (create a name for) specific layers that you should use:
docker run -it 45b3c59b9130 bash
Repositories are constructed this way because whenever an image builder creates a new image, the differences are saved as a layer. There are two main ways that new layers are created in a repository. First, if building an image manually, each “commit” creates a new layer. If the image builder is building an image with a Dockerfile, each directive in the file creates a new layer. It is useful to have visibility into what has changed in a container repository between each layer.
Tag
Even though a user can designate that a container mount and start from any layer in a repository, they shouldn’t necessarily do that. When an image builder creates a new repository, they will typically label the best image layers to use. These are called tags and are a tool for container image builders to communicate to container image consumers which layers are best to consume. Typically, tags are used to designate versions of software within in the repository. This is by convention only – in reality, the OCI nor any other standard mandates what tags can be used for, and they can be abused for anything that a user wants. Be careful dong this because it can create a lot of confusion in development, operations and architecture teams, so document it it well if you use it for anything other than software version.
There is one special tag – latest – which typically points to the layer containing the latest version of software in the repository. This special tag still just points to an image layer, like any other tag, so it can be abused.
To remotely view the tags available in a repository, run the following command (the jq utility makes the output a lot more readable):
curl -s registry.access.redhat.com/v1/repositories/rhel7/tags | jq
{
“7.0-21”: “e1f5733f050b2488a17b7630cb038bfbea8b7bdfa9bdfb99e63a33117e28d02f”,
“7.0-23”: “bef54b8f8a2fdd221734f1da404d4c0a7d07ee9169b1443a338ab54236c8c91a”,
“7.0-27”: “8e6704f39a3d4a0c82ec7262ad683a9d1d9a281e3c1ebbb64c045b9af39b3940”,
“7.1-11”: “d0a516b529ab1adda28429cae5985cab9db93bfd8d301b3a94d22299af72914b”,
“7.1-12”: “275be1d3d0709a06ff1ae38d0d5402bc8f0eeac44812e5ec1df4a9e99214eb9a”,
“7.1-16”: “82ad5fa11820c2889c60f7f748d67aab04400700c581843db0d1e68735327443”,
“7.1-24”: “c4f590bbcbe329a77c00fea33a3a960063072041489012061ec3a134baba50d6”,
“7.1-4”: “10acc31def5d6f249b548e01e8ffbaccfd61af0240c17315a7ad393d022c5ca2”,
“7.1-6”: “65de4a13fc7cf28b4376e65efa31c5c3805e18da4eb01ad0c8b8801f4a10bc16”,
“7.1-9”: “e3c92c6cff3543d19d0c9a24c72cd3840f8ba3ee00357f997b786e8939efef2f”,
“7.2”: “6c3a84d798dc449313787502060b6d5b4694d7527d64a7c99ba199e3b2df834e”,
“7.2-2”: “58958c7fafb7e1a71650bc7bdbb9f5fd634f3545b00ec7d390b2075db511327d”,
“7.2-35”: “6883d5422f4ec2810e1312c0e3e5a902142e2a8185cd3a1124b459a7c38dc55b”,
“7.2-38”: “6c3a84d798dc449313787502060b6d5b4694d7527d64a7c99ba199e3b2df834e”,
“latest”: “6c3a84d798dc449313787502060b6d5b4694d7527d64a7c99ba199e3b2df834e”
}
Repository
When using the docker command, a repository is what is specified on the command line, not an image. In the following command, “rhel7” is the repository.
docker pull rhel7
This is actually expanded automatically to:
docker pull registry.access.redhat.com/rhel7:latest
This can be confusing, and many people refer to this as an image or a container image. In fact, the docker images sub-command is what is used to list the locally available repositories. Conceptually, these repositories can be thought of as container images, but it’s important to realize that these repositories are actually made up of layers and include metadata about in a file referred to as the manifest (manifest.json):
docker images
REPOSITORY TAG IMAGE ID CREATED VIRTUAL SIZE
registry.access.redhat.com/rhel7 latest 6883d5422f4e 4 weeks ago 201.7 MB
registry.access.redhat.com/rhel latest 6883d5422f4e 4 weeks ago 201.7 MB
registry.access.redhat.com/rhel6 latest 05c3d56ba777 4 weeks ago 166.1 MB
registry.access.redhat.com/rhel6/rhel latest 05c3d56ba777 4 weeks ago 166.1 MB
…
When we specify the repository on the command line, the Container Engine is doing some extra work for you. In this case, the docker daemon (not the client tool) is configured with a list of servers to search. In our example above, the daemon will search for the “rhel7” repository on each of the configured servers.
In the above command, only the repository name was specified, but it’s also possible to specify a full URL with the docker client. To highlight this, let’s start with dissecting a full URL.

Another way you will often see this specified is:
REGISTRY/NAMESPACE/REPOSITORY[:TAG]
The full URL is made up of a standard server name, a namespace, and optionally a tag. There are actually many permutations of how to specify a URL and as you explore the docker ecosystem, you will find that many pieces are optional. The following commands are all valid and all pull some permutation of the same repository:
docker pull registry.access.redhat.com/rhel7/rhel:latest
docker pull registry.access.redhat.com/rhel7/rhel
docker pull registry.access.redhat.com/rhel7
docker pull rhel7/rhel:latest
Namespace
A namespace is a tool for separating groups of repositories. On the public DockerHub, the namespace is typically the username of the person sharing the image, but can also be a group name, or a logical name.
Red Hat uses the namespace to separate groups of repositories based on products listed on the Red Hat Federated Registry server. Here are some example results returned by registry.access.redhat.com. Notice, the last result is actually listed on another registry server. This is because Red Hat works to also list repositories on our partner’s registry servers:
registry.access.redhat.com/rhel7/rhel
registry.access.redhat.com/openshift3/mongodb-24-rhel7
registry.access.redhat.com/rhscl/mongodb-26-rhel7
registry.access.redhat.com/rhscl_beta/mongodb-26-rhel7
registry-mariadbcorp.rhcloud.com/rhel7/mariadb-enterprise-server:10.0
Notice, that sometimes the full URL does not need to be specified. In this case, there is a default repository for a given namespace. If a user only specifies the fedora namespace, the latest tag from the default repository will be pulled to the local server. So, running the following commands is essentially the same, each one more specific:
docker pull fedora
docker pull docker.io/fedora
docker pull docker.io/library/fedora:latest
Kernel Namespace
A kernel namespace is completely different than the namespace we are referring to when discussing Repositories and Registry Servers. When discussing containers, Kernel namespaces are perhaps the most important data structure, because they enable containers as we know them today. Kernel namespaces enable each container to have it’s own mount points, network interfaces, user identifiers, process identifiers, etc.
When you type a command in a Bash terminal and hit enter, Bash makes a request to the kernel to create a normal Linux process using a version of the exec() system call. A container is special because when you send a request to a container engine like docker, the docker daemon makes a request to the kernel to create a containerized process using a different system call called clone(). This clone() system call is special because it can create a process with its own virtual mount points, process ids, user ids, network interfaces, hostname, etc
While, technically, there is no single data structure in Linux that represents a container, kernel namespaces and the clone() system call are as close as it comes.
Graph Driver
When the end user specifies the Tag of a container image to run – by default this is the latest Tag – the graph driver unpacks all of the dependent Image Layers necessary to construct the data in the selected Tag. The graph driver is the piece of software that maps the necessary image layers in the Repository to a piece of local storage. The container image layers can be mapped to a directory using a driver like Overlay2 or in block storage using a driver like Device Mapper. Drivers include: aufs, devicemapper, btrfs, zfs, and overlayfs.
When the container is started, the image layers are mounted read-only with a kernel namespace. The Image Layers from the Repository are always mounted read only but by default, a separate copy-on-write layer is also set up. This allows the containerized process to write data within the container. When data is written, it is stored in the copy-on-write layer, on the underlying host. This copy-on-write layer can be disabled by running the container with an option such as –readonly.
The docker daemon has it’s own set of Graph Drivers and there are other open source libraries which provide Graph Drivers such as containers/images which is used in tools like CRI-O, Skopeo and other container engines.

Determining which graph driver you are using can be done with the docker info command:
[root@rhel7 ~]# docker info
…
Storage Driver: devicemapper
Pool Name: docker-253:1-884266-pool
Pool Blocksize: 65.54 kB
Backing Filesystem: xfs
Data file: /dev/loop0
Metadata file: /dev/loop1
Data Space Used: 3.037 GB
Data Space Total: 107.4 GB
Data Space Available: 2.56 GB
Metadata Space Used: 2.707 MB
Metadata Space Total: 2.147 GB
Metadata Space Available: 2.145 GB
Udev Sync Supported: true
Deferred Removal Enabled: false
Data loop file: /var/lib/docker/devicemapper/devicemapper/data
Metadata loop file: /var/lib/docker/devicemapper/devicemapper/metadata
Library Version: 1.02.107-RHEL7 (2015-10-14)
There are many types of Container design patterns forming. Since containers are the run time version of a container image, the way it is built is tightly coupled to how it is run.
Some Container Images are designed to be run without privilege, while others are more specialized and require root-like privileges. There are many dimensions in which patterns can be evaluated and often users will see multiple patterns or use cases tackled together in one container image/container.
This section will delve into some of the common use cases that users are tackling with containers.
Application Containers
Application containers are the most popular form of container. These are what developers and application owner’s care about. Application containers contain the code that developers work on. They also include things like MySQL, Apache, MongoDB, and or Node.js.
There is a great ecosystem of application containers forming. Projects like Software Collections are providing secure and supportable applications container images for use with Red Hat Enterprise Linux. At the same time, Red Hat community members are driving some great cutting edge applications containers.
Red Hat believes that Application Containers should not typically require special privileges to run their workloads. That said, production container environments typically require much more than just non-privileged application containers to provide other supporting services.
Operating System Containers
See also System Containers
Operating System Containers are containers which are treated more like a full virtual operating system. Operating System Containers still share a host kernel, but run a full init system which allows them to easily run multiple processes. LXC and LXD are examples of Operating System Containers because they are treated much like a full virtual machine.
It is also possible to approximate an Operating System Container with docker/OCI based containers, but requires running systemd inside the container. This allows an end user to install software like they normally would and treat the container much more like a full operating system.
yum install mysql
systemctl enable mysql
This makes it easier to migrate existing applications. Red Hat is working hard to make Operating System Containers easier by enabling systemd to run inside a container and by enabling management with machined. While many customers aren’t (yet) ready to adopt micro-services, they can still gain benefits from adopting image based containers as a software delivery model.
Pet Containers
While Red Hat certainly recommends, supports and evangelizes the use cloud native patterns for new application development, in reality not all existing applications will be rewritten to take advantage of new patterns. Many existing applications are one of a kind, and one of kind applications are often referred to as Pets. Containers built specifically to handle these pet applications are sometimes referred to as Pet Containers
Pet containers provide users with the portability and convenience of a standardized container infrastructure relying on registry servers, container images, and standard container hosts for infrastructure, but provide the flexibility of a traditional environment within the container. The idea is to make things easier to containerize existing applications, such as using systemd in a container. The goal is to reuse existing automation, installers, and tools to easily create a container image that just runs.
Super Privileged Containers
When building container infrastructure on dedicated container hosts such as Red Hat Enterprise Linux Atomic Host, systems administrators still need to perform administrative tasks. Whether used with distributed systems, such as Kubernetes or OpenShift or standalone container hosts, Super Privileged Containers (SPC) are a powerful tool. SPCs can even do things like load specialized kernel modules, such as with systemtap.
In an infrastructure that is built to run containers, administrators will most likely need SPCs to do things like monitoring, backups, etc. It’s important to realize that there is typically a tighter coupling between SPCs and the host kernel, so administrators need to choose a rock solid container host and standardize on it, especially in a large clustered/distributed environment where things are more difficult to troubleshoot. They then need to select a user space in the SPC that is compatible with the host kernel.
Tools & Operating System Software
Linux distributions have always provided users with system software such as Rsyslogd, SSSD, sadc, etc. Historically, these pieces of system software were installed through RPM or DEB packages. But with the advent of containers as a packaging format, it has become both convenient and easy to install system software through containers images. Red Hat provides some pre-packaged containers for things like the Red Hat Virtualization tools, rsyslog, sssd, and sadc.
New design patterns are forming as more and more people deliver software with containers. Red Hat engineering is leveraging and driving many of these patterns in the community. The goal of this section is to help highlight and define some of these patterns.
The way a container is saved on disk (i.e. its image format) can have a dramatic affect on how it is run. For example, a container which is designed to run sssd needs to have special privileges whenever it’s run, or it can’t do its job. The following is a short list of patterns that are forming in the container community:
Application Images
These images are what end users consume. Use cases range from databases and web servers, to applications and services buses. These can be built in house or delivered to a customer from an ISV. Often end users will investigate and care about what bits were used to create a standalone image. Standalone images are the easiest kind of image to consume, but the hardest to design, build, and patch.
Base Images
A base image is one of the simplest types of images, but you will find a lot of definitions. Sometimes users will refer to corporate standard build, or even an application image as the “base image.” Technically this is not a base image. These are Intermediate images.
Simply put, a base image is an image that has no parent layer. Typically, a base image contains a fresh copy of an operating system. Base images normally include the tools (yum, rpm, apt-get, dnf, microdnf) necessary to install packages / make updates to the image over time. While base images can be “hand crafted”, in practice they are typically produced and published by open source projects (like Debian, Fedora or CentOS) and vendors (like Red Hat). The provenance of base images is critical for security. In short, the sole purpose of a base image is to provide a starting place for creating your derivative images. When using a dockerfile, the choice of which base image you are using is explicit:
FROM registry.access.redhat.com/rhel7-atomic
Builder Images
These are a specialized form of container image which produce application container images as offspring. They include everything but a developer’s source code. Builder images include operating system libraries, language runtimes, middleware, and the source-to-image tooling.
When a builder image is run, it injects the developers source code and produces a ready-to-run offspring application container image. This newly created application container image can then be run in development or production.
For example, if a developer has PHP code and they want to run it in a container, they can use a PHP builder image to produce a ready to run application container image. The developer passes the GitHub URL where the code is stored and the builder image does the rest of the work for them. The output of a Builder container is an Application container image which includes Red Hat Enterprise Linux, PHP from Software Collections, and the developer’s code, all together, ready to run.
Builder images provide a powerful way to go from code to container quickly and easily, building off of trusted components.
Containerized Components
A container is meant to be deployed as part of a larger software system, not on its own. Two major trends are driving this.
First, microservices are driving the use of best of breed components – this is also driving the use of more components combined together to build a single application. Containerized components are meeting the need to deploy an expanding quantity of complex software more quickly and easily. Each of these components can have different revisions, and container images help enable this. Application definitions such as Kubernetes/OpenShift deployments yaml/json, open service broker, OpenShift Templates, and Helm Charts are all making it possible to define applications at a higher level.
Second, not all pieces of software are easy to deploy as containers. Sometimes, it makes sense to containerize only certain components which are easier to move to containers or provide more value to the overall project. With multi-service application, some services may be deployed as containers, while others may be deployed through traditional a traditional methodology such as an RPM or installer script – see Pet Containers. But, other components might be difficult to put into containers because they are too tightly coupled to break up, need access to special hardware, or perhaps requires lower level kernel APIs, etc. Within a larger application there will likely be parts of the application that can be containerized, and parts that can’t. Containerized components represent the parts that can and are containerized. Containerized components are intended to be ran as part of a specific application, not standalone. It’s important to understand that containerized components are not designed to function on their own. They provide value to a larger piece of software, but provide very little value on their own.
For example, when OpenShift Enterprise 3.0 was released, most of the core code was deployed using RPMs, but after installation administrators deployed the router and registry as containers. With the release of OpenShift 3.1 an option was added to the installer to deploy the master, node, openvswitch and etcd components as containers – after installation, administrators were given the option to deploy elasticsearch, fluentd, and kibana as containers.
While the OpenShift installer still makes modifications to a server’s file system, all of the major software components can now be installed using container images. What makes these containerized components is that, for example, an instance of the etcd image built into OpenShift should and would never be used to store data for your customer facing application code, because it is a containerized component designed to be run as part of OpenShift Container Platform.
With the latest releases of OpenShift, there is a trend towards more and more containerized components. The containerized component pattern is becoming more and more common and other software vendors are seeing an advantage to deploying as containerized components.
Deployer Images
A deployer image is a specialized kind of container which, when run, deploys or manages other containers. This pattern enables sophisticated deployment techniques such as mandating the start order of containers, or first run logic such as populating schema or data.
As an example, the “image/container type” pattern is used to deploy the logging and metrics in OpenShift. Deploying these components with a deployer container allows the OpenShift engineering team to control start order of the different components and make sure they are all up and running together.
Intermediate Images
An Intermediate image is any container image that relies on a base image. Typically, core builds, middleware and language runtimes are built as layers on “top of” a base image. These images are then referenced in the FROM directive of another image. These images are not used on their own, they are typically used as a building block to build a standalone image.
It is common to have different teams of specialists own different layers of an image. Systems administrators may own the core build layer, while “developer experience” may own the middleware layer. Intermediate Images are built to be consumed by other teams building images, but can sometimes be run standalone too, especially for testing.
Intermodal Container Images
Intermodal container images, analogous to intermodal shipping containers, are images that have hybrid architectures. For example, many Red Hat Software Collections images can be used in two ways. First, they can be used as simple Application Containers running a fully contained Ruby on Rails and Apache server. Second, they can be used as Builder Images inside of OpenShift Container Platform. In this case, the output child images which contain Ruby on Rails, Apache, and the application code which the source to image process was pointed towards during the build phase.
The intermodal pattern is becoming more and more common to solve two business problems with one container image.
System Containers
When system software is distributed as a container, it often needs to run super privileged. To make this deployment easier, and to allow these containers to start before the container runtime or orchestration, Red Hat developed a special container pattern called System Containers. System Containers start early in the boot process and rely on the atomic command and systemd to be started independent of any container runtime or orchestration. Red Hat provides System Containers for many pieces of software including rsyslog, cockpit, etcd, and flanneld. In the future, Red Hat will expand the list.
This design pattern will make it easier for administrators to add these services to Red Hat Enterprise Linux and Atomic Host in a modular way.
Containers are quite easy to consume, but when building a production container environment, it shifts the complexity behind the scenes. To be able to discuss architectures, and how you will build your environment, it’s important to have shared nomenclature. There are a lot of pitfalls as you dig deeper into building and architecting your environment. We leave you with a couple of critical ones to remember.
People often use the words container image and repository interchangeably and the docker sub-commands don’t make a distinction between an image and a repository. The commands are quite easy to use, but once architecture discussions start, it’s important to understand that a repository is really the central data structure.
It’s also quite easy to misunderstand the difference between a namespace, repository, image layer, and tag. Each of these has an architectural purpose. While different vendors, and users are using them for different purposes, they are tools in our toolbox.

The goal of this article is to leave you with the ability to command this nomenclature so that more sophisticated architectures can be created. For example, imagine that you have just been charged with building an infrastructure that limits, based on role, which namespaces, repositories, and even which image layers and tags can be pushed and pulled based on business rules. Finally, remember that how a container image is built will have profound effect on how it is to be run (orchestrated, privileged, etc).
For further reading, check out the Architecting Containers series:
As always, if you have comments or questions, please leave a message below.
Source